Here are the 3d design files, PCB files, links to code, etc.
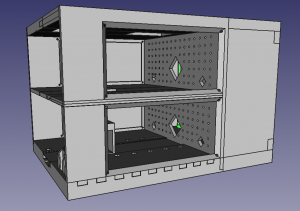
Note: these were all made “for my own use”: In particular the 3d models are really awkwardly put together (first time I played with freecad).
The circuit/PCB includes components for:
- measuring the battery voltages
- driving the stepper motor (to turn the platform).
- controlling and triggering the camera/flashes
It is likely most people only want the last of these.
The logic here is that a trigger is sent to the camera. The camera itself has a ‘flash trigger’ it sends back. I then AND this (using a 7408 quad-AND chip) with four flash-selection signals, from the pi. This is because I wanted to control which flashes fired. These then operate 4 transistors which trigger the four flashes.
The best way to approach using the tracking idea, is probably to just build your own solution from scratch, using the general principles:
- A global-electronic shutter, so that you can adjust the exposure to match the flash
- Subtracting frames, and then using a bit of logic to try to remove false-positives:
- Most FPs seem to be ‘blurry’ large blobs (the tags, if the camera is focused are sharp points).
PCB layout - Consider only considering a true-positive if you have seen a bee in 2-3 images in the same part of the image.
- Most FPs seem to be ‘blurry’ large blobs (the tags, if the camera is focused are sharp points).
- Triggering – I use a transistor to do this. I wonder if there are modules that convert from a pi’s GPIO to trigger a flash…
I’m afraid I’ve not looked into raspberry’s new global electronic shutter camera. But this sounds really like a good direction in future: Currently there’s a lot of faff and low reliability around dealing with the cameras I used (the ethernet connection to the camera, the annoying hirose connector, the 12V+ the camera needs etc).
Files:
- pcb/circuit schematic: link. (created with kicad).
- 3d model of tracking system: link. (created with freecad).
- The bee_track python module is on github. See README for install notes. link.